What do you stand to gain from outstanding data hygiene?
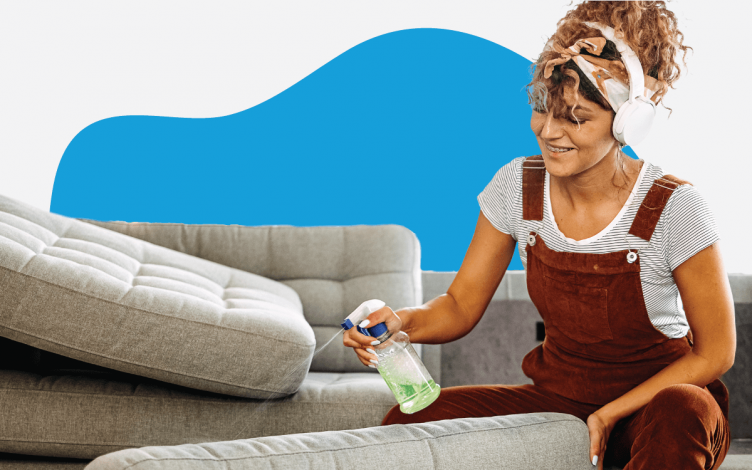
Dirty data is a real difficulty that’s only getting worse. Everyday people generate 2.5 quintillion bytes of data. That’s 2,500,000,000,000,000,000 bytes of data created just by sending emails, texts, searching for information or creating content1 — things we do without thinking everyday. The entire digital universe has over 44 zettabytes of data.2 In 2023 we’re generating three times the amount of data we generated in 2019.
Dirty data is data that includes duplicates, is outdated, insecure, incomplete, inaccurate, or inconsistent in some other way. Misspelled email addresses, missing fields, duplicate entries and outdated information like phone numbers are all examples of dirty data. When you’re sending a lone email to your Aunt, it’s easy enough to call her up to check to make sure you have the right address. But when you’re dealing with thousands and thousands of entries, it’s not easy at all.
That’s why incorporating clean data strategies and systems into your current structure can feel daunting. But you have more to gain than to lose–if dirty data is any one thing, it’s a waste of money. And you’ll gain better targeting, better fraud detection, and better risk management.
You’ll target the right people
Personalize, personalize, personalize. Marketers will tell you that customers respond to personalized material 80%3 better than ineffective “spray and pray” techniques. Discounts that actually apply to the customer you’re sending them to have a better shot at getting the customer in the door, for instance. All of that personalization work that your team has been doing goes away in the blink of an eye if it doesn’t even reach the right email address because of a misspelling or an outdated file. Or, If your lead list has duplicates, you’re spending twice as much per duplicated customer as you think, but only getting half the results.
Clean data also can help you fill in the gaps so you can create meaningful customer modeling. Oftentimes, a data service provider can not only provide email addresses to match your mailing addresses (or vice versa) but can also provide psychographic or firmographic data so you can see the whole customer. Then, the data can be organized to show you new audience segments you may not have seen before.
Case Study
Data Axle worked with an automotive retailer recently to clean up their data. The company was worried about sending their customers duplicate messaging, or sending incorrect messaging to customers, both of which would result in a poor customer experience and jeopardize the company’s reputation.
So Data Axle worked with the retailer to implement a data hygiene solution that would ensure the right information always went to the right people through targeted marketing campaigns–duplicate listings would be found and “matched” together, and information on each customer would be matched with verified information to ensure the company’s list was up to date and complete. The new system also gave the company a chance to analyze and find areas where they could improve.
Ultimately, because they were able to rely on their data and demographic information, they were able to assess their options and place a new store in an area with their ideal customer base.
What can you do to start ensuring information coming in is accurate?
- Ask for email addresses twice to weed out misspellings
- Ask customers to provide their updated information
- Ask customers simple preference questions to gauge engagement, personalize messaging for that specific customer and add to your customer data sets to improve your targeting overall
You’ll stop fraud in its tracks
Let’s say a 50 year old man tries to take out a credit card using the stolen SSN he recently acquired for a 20 year old college student a thousand miles away. He has all the information he needs for birthdays and previous addresses– but your system has already matched his address to a 50 year old male, not a 20 year old college student. Something’s wrong, and your system can pick up on it if it has good, clean data.
Data matching or data enhancement can help detect and prevent fraud like this. The company’s customer data on file is matched and appended to a verifiable third party source. This allows companies to find potential discrepancies in the mass amounts of data by creating a more accurate prediction of a customer profile. When you have high data match rates, you know your information is accurate, and you can provide your customers with better security and protection when they interact with your product or service.
Predictive modeling can also reduce fraud by scoring your system’s likelihood for producing fraud, and scoring new applications for signs of fraud so you can have a specialist look into those applications further. As of 2019, the Certified Fraud Examiners found that only 13% of organizations used machine learning to detect fraud.4
Now, in 2023, an estimated 58% of the financial services industry alone uses AI to detect fraud.5 By understanding each user’s usual transaction rates, AI can flag sharp increases in purchases or transfers, or flag a purchase made that doesn’t ship to the card owner’s country.
What can you do now to start reducing fraud?
- Standardize your data so that you and your system can identify patterns quicker
- Ensure your data is up to date and thoroughly verified before it enters your system
- If you’re not a fraud or data specialist, ask for help!
You’ll make better decisions and mitigate risk
If you’re working with poor inboxing rates, undeliverable direct mail, or low data match rates, then you can’t really know what your customers are responding well to. Maybe you sent out ten thousand flyers, but five thousand of those went to out of date addresses or bounced back. If you get two thousand responses, you might think your response rate is 20%. But actually it’s much higher than that. How can you make a sound financial or strategic decision if you don’t know that half of your list never saw your material?
Identifying and correcting errors is a major part of data hygiene, and it’s a continually evolving process. There’s 44 zettabytes of data out there, after all!
Insurance organizations are finding other ways to double check their data and assess risk, too. Companies are encouraging employees to use wearable fitness tech to track their health in a number of different ways. With this kind of information, health insurance companies have a clearer idea of the risk a group of employees represents, and can offer discounts. Not every employee is comfortable with this kind of insight into their private health, but with data matching, insurance groups can compare health spend rates of similar employee groups to get a better understanding of risks.
What can you do now to start mitigating risk?
- Clean up your data to get rid of dead weight information
- Organize and append your data to show you new segments you couldn’t see before
- Double check new data coming in with verified third party lists
It’s a challenge to maintain accurate data. Customers’ names change and they switch addresses. Businesses change ownership or operating status. Clean, accurate data (and the systems that verify and maintain that accuracy) allows you to make meaningful insights, prevent costly mistakes, and save time.
It’s easier than ever to obtain and maintain data. Data Axle has a powerful mix of data sets that target a plethora of business needs that’s accessible through your instance of Snowflake. You can facilitate the processing of accurate and clean consumer and business records on a monthly, quarterly, weekly, or even daily basis. Learn more here.
1 https://www.g2.com/articles/big-data-statistics
2 https://blog.gitnux.com/big-data-statistics/
3 https://www.epsilon.com/us/about-us/pressroom/
4 https://www.prnewswire.com/news-releases/study-ai-for-fraud-detection-to-triple-by-2021-300872958.html
5 https://www.inscribe.ai/fraud-detection/ai-fraud-detection